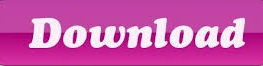
Outfile_name = i.replace(".png", ".npy") # "xyz.npy" and "xty.

Pillow image resize code#
T = np.round(t.clip(0, 255)).astype(np.uint8) # Convert from float to uint8 (use rounding and clipping for "cleaner" conversion).Ĭomplete code sample (including the loop): import numpy as np T = norm_t*std + mean # Unnormalized (get the original pixels before normalizing) Mean, std = tuple(mean_std) # Get mean and std as two scalars Mean_std = np.load(f) # Load mean and std (that were saved before). Norm_t = np.load(f) # Load normalized array Np.save(f, np.array()) # Save mean and std as 2 elements numpy array after the "pixels" array (in the same file).Įxample for loading the normalized image with the mean and std, and restoring and showing the original image: with open(outfile_name, 'rb') as f: Np.save(f, pixels) # Save the normalized image. Saving and restoring the normalized image with the original mean and std:Įxample for saving pixels with mean and std: with open(outfile_name, 'wb') as f: img Image.open ('./myimage.png') img.show () Crop the image. Adding Image.show () displays the image in an external viewer. Change the crop size according your need. To crop an image with Pillow: Import Pillow’s Image class: from PIL import Image Load an image from the file system and, with the Image.open () class, convert the image into an instance of the Image class. For example, the given size is (300,350) for rectangular crop and 250 for square crop. img Image.open('lounge.jpg') Define a transform to resize the image to a given size. We may display the normalized image like this (for example): omarray(((t-t.min())/(t.max()-t.min())*255).astype(np.uint8), 'RGB').show()īy the way you are asking your question, it looks like restoring the original image is a better solution. The input image is a PIL image or a torch tensor or a batch of torch tensors. When loading the normalized image, also load mean and std - that allows us to restore the original image (before normalizing). I think your best option is to save the mean and the std with each normalized image (in the same. I searched lots of documents and gave many hours to solve it.I am waiting any kind of helps. I tried to save them as array: np.save(outfile, pixels) # print('Mean: %.3f, Standard Deviation: %.3f' % (mean, std)) # calculate global mean and standard deviation "/content/drive/MyDrive/Colab Notebooks/images/evre1/xty.png"] How can I save and load them? files = ["/content/drive/MyDrive/Colab Notebooks/images/evre1/xyz.png", But I couldn't find a way to save images after making changes below. It will return the resized image and also print the size of the original image and the output image on the console.I want to normalize my images and use it in the training.

# define transformt o resize the image with given size Print("Size of the Original image:", size) # compute the size(width, height) of image The original image is of size (700,700) # import the required libraries The input image is resized to (300, 350). This image is used as the input file in all the following examples. Change the crop size according your need.Īpply the above-defined transform on the input image to resize the input image. The input image is a PIL image or a torch tensor or a batch of torch tensors.ĭefine a transform to resize the image to a given size.

Make sure you have already installed them.
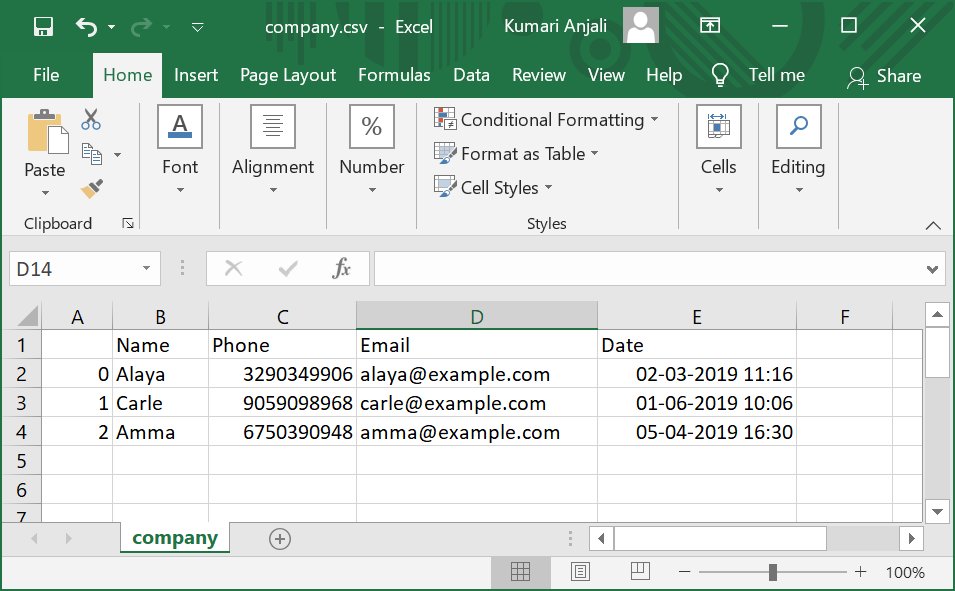
In all the following examples, the required Python libraries are torch, Pillow, and torchvision. We could use the following steps to resize an input image to a given size. It returns a resized image of given size. If size is an int, then the resized image will be a square image. size is a sequence like (h, w), where h and w are the height and width of the output image. Size – Size to which the input image is to be resized. If the image is neither a PIL image nor a tensor image, then we first convert it to a tensor image and then apply the Resize()transform. This transform also accepts a batch of tensor images, which is a tensor with where B is the number of images in the batch. A tensor image is a torch tensor with shape, where C is the number of channels, H is the image height, and W is the image width. Resize() accepts both PIL and tensor images. It's one of the transforms provided by the ansforms module. The Resize() transform resizes the input image to a given size.
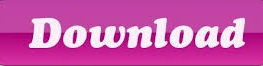